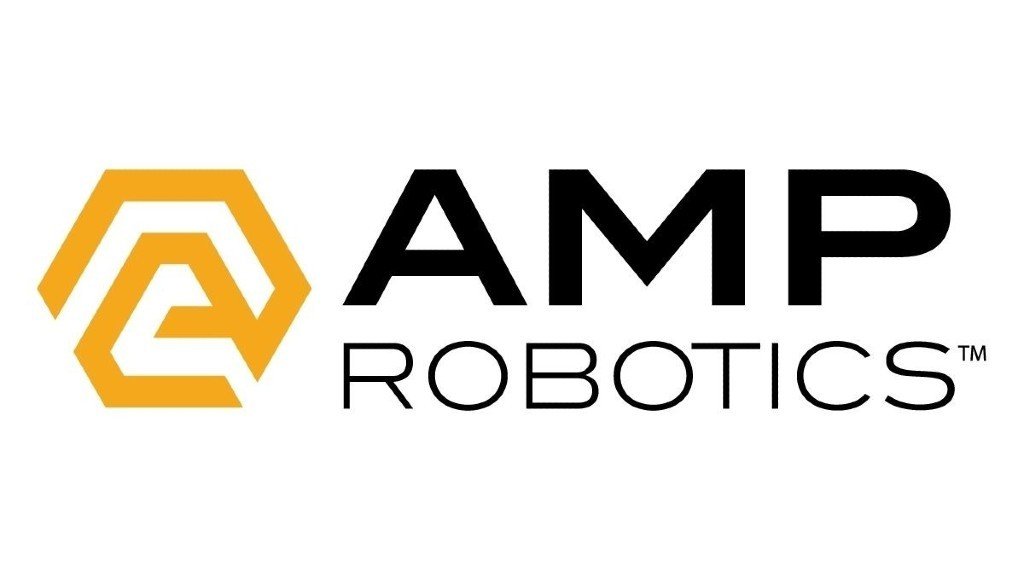
AMP’s artificial intelligence (AI) platform AMP NeuronTM uses cameras to scan mixed waste streams and identify the different materials. Neuron’s deep learning capability allows for continuous improvement of the identification and categorisation of paper, plastics and metals, by colour, size, shape, brand and other traits.
AMP CortexTM is the body to AMP Neuron's brain. Cortex is a high-speed intelligent robotics system that performs the physical task of sorting, picking and placing material based on information fed by the ‘eyes and brain’ of AMP Neuron. Cortex can sort recyclables at a rate of 80 items per minute with an accuracy of up to 99%.
AMP Neuron encompasses the largest known real-world dataset of recyclable materials for machine learning, with the ability to classify more than 100 different categories and characteristics of recyclables across single-stream recycling, e-scrap and construction and demolition debris, and reaching an object recognition run rate of more than 10 billion items annually.
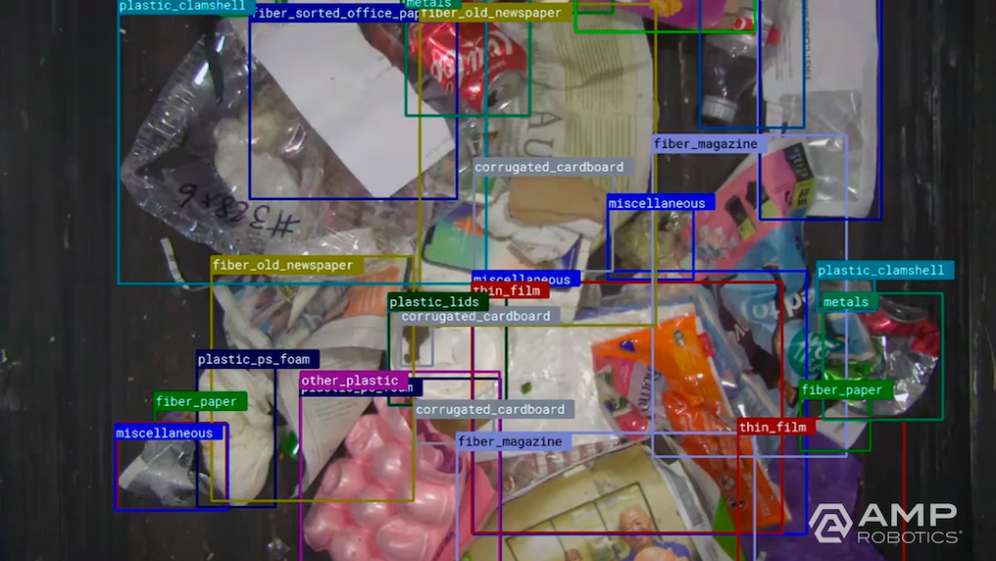
Why it's an example of a circular economy
Globally, AMP has estimated that more than USD 200 billion worth of recyclable materials goes unrecovered annually. The economics and efficiency of identifying and sorting paper, plastics, metals, and other recyclables from the waste stream creates a major challenge for material recovery. In recent years, the waste industry has also faced stricter international quality standards for contamination-free imports of recyclable materials, leaving the industry in search of cost-effective ways to meet these requirements.
AMP’s technologies allow more recyclables to be captured from waste streams, producing a greater volume of high-purity secondary resources. In one recycling centre in Virginia that installed the technology, the volume of recycled material increased by 10%.
The job of material sorting, which is typically carried out by humans, is physically demanding, poses safety risks and is prone to human error. Having humans and robots working side by side, can stabilise the workforce and create improvements in the quality of full-time jobs. In the Virginia plant, the capital cost of the technology was offset through a reduction in recruitment and training costs of hard-to-find temporary workers.
AI-enabled recycling is scaling fast
In a sign of the proliferation of AI-driven recycling, AMP partnered with Waste Connections to deploy 25 robots across its materials recovery facilities nationwide, the company’s largest order to date.
AMP’s reach extends into other areas of the circular economycircular economyA systems solution framework that tackles global challenges like climate change, biodiversity loss, waste, and pollution. It is based on three principles, driven by design: eliminate waste and pollution, circulate products and materials (at their highest value), and regenerate nature.; one of its first corporate partners is Keurig Dr Pepper (KDP). The companies worked together to equip AMP’s robotics systems to properly identify and sort K-Cup coffee pods in recycling facilities. AMP’s installation at Evergreen, one of the largest recyclers of PET bottles in the US, demonstrates the company’s continuous market expansion with plastics reclaimers in addition to its infrastructure modernisation efforts with materials recovery facilities.
Benefits
Applying AI and automation to waste sorting offers opportunities to improve performance and reduce operating costs, increasing the value of the secondary resources and improving the economics of recycling.
The data that is captured by AMP’s technology can be used to save time and lower costs across many areas of a materials recovery facility by:
Avoiding costly rejections by validating contamination-free material for resale
Eliminating error-prone manual monitoring processes
Preventing system downtime by anticipating issues
Spotting hazards that could cause physical risks to staff
Identifying volume and material composition trends to optimise operations
Further information
The Ellen MacArthur Foundation’s Artificial Intelligence and the Circular Economy report